top of page

Canaria Technologies Blog
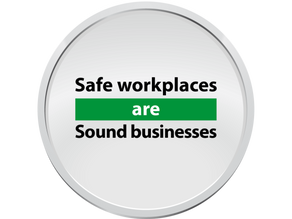
Canaria Technologies
Nov 1, 20232 min read
Mining Safety Violations Out of Control
According to the US Department of Labor, September impact inspections at 9 mines in 7 states identified more than 120 safety, health...
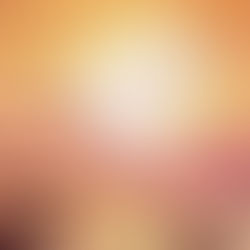
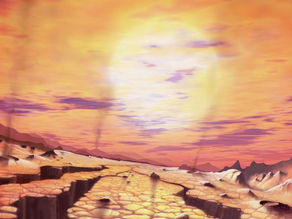
Canaria Technologies
Oct 19, 20233 min read
Overcome by Heat
‘I Dropped To The Ground And Passed Out’: Grant And Chezzi Denyer Eliminated From The Amazing Race Australia After Shock Medical Incident...
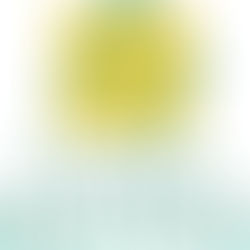
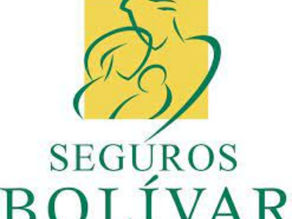
Canaria Technologies
Sep 29, 20231 min read
Wearing the Canaria-V for Risk Mitigation: Safe Work in Extreme Environments
The #PreventionForum2023 is here! Charly WIGSTROM BRONCHEUR, Neylla Marcela Avila Florez, M.Sc and Sergio Valencia Krauss will be some of...
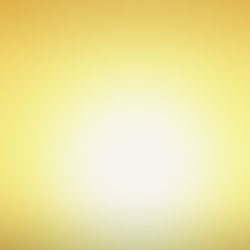
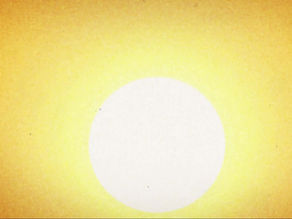
Canaria Technologies
Aug 22, 20233 min read
CAUTION: Heat Stress Preparedness for Workers
Canaria Technologies has proven wearable technology to mitigate heat stress and cognitive fatigue in extreme environments. Contact us
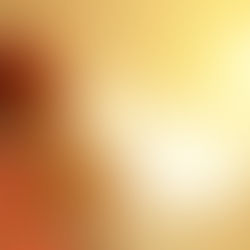
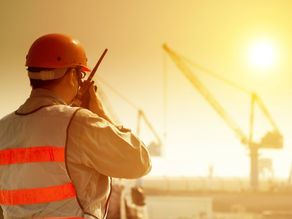
Canaria Technologies
Jul 25, 20231 min read
Working in Outdoor and Indoor Heat Environments
According to Occupational Safety and Health Administration(OSHA), Millions of workers are exposed to heat in their workplaces. Although...
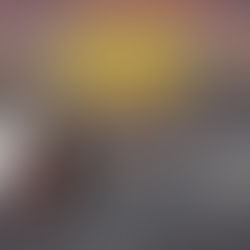
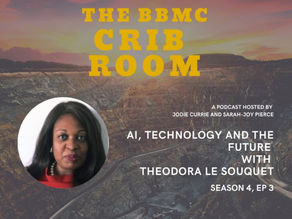
theodora410
Jul 12, 20231 min read
AI, Technology and the Future
Don't miss Episode 4 of this season's BBMC Crib Room podcast with Theodora Le Souquet, Managing Director of Canaria Technologies - a...
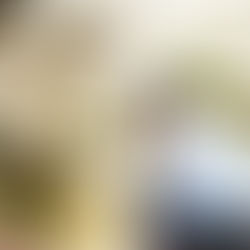
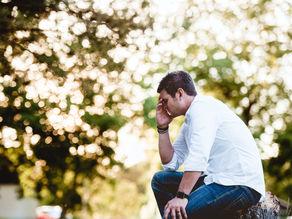
Canaria Technologies
Mar 1, 20231 min read
Why heat and fatigue?
Heat stress and cognitive fatigue are two interrelated phenomena that can have a significant impact on an individual's cognitive...
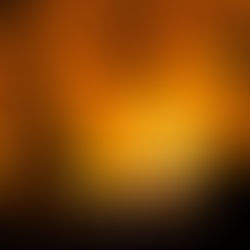
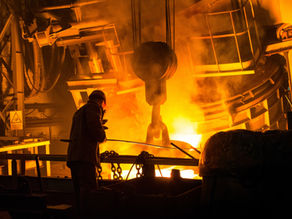
Canaria Technologies
Feb 16, 20232 min read
Mitigating Heat Stress in Mining
Heat stress is a common occupational hazard in mining, particularly in underground mines where the ambient temperature can be...
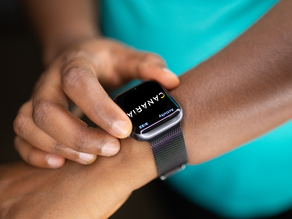
-
Nov 25, 20227 min read
What is PPG Technology and How Does it Work?
Wearable technology has increased in popularity since the advent of smartwatches and fitness trackers.
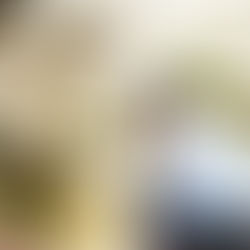
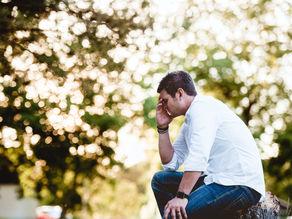
-
Oct 10, 20225 min read
What is Cognitive Fatigue - Signs, Symptoms and Management
Cognitive Fatigue is a common issue in our everyday life, defined as a psychobiological state.
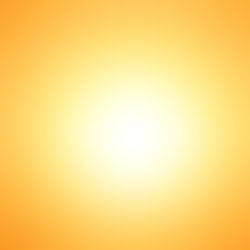
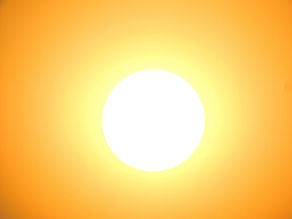
-
Sep 1, 20225 min read
Heat Stress - Signs, Symptoms and Protocols for Workplace Health and Safety Management
Heat-related illnesses can be fatal if prompt medical attention is not sought, especially in cases of heat stroke.
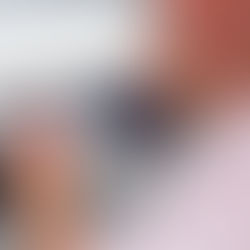
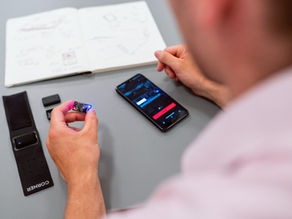
-
Aug 12, 20224 min read
Smart PPE - the Next Generation of Risk-Prevention Technology
Smart PPE is the next generation of risk-prevention technology, which combines traditional methods of protection with enhanced materials.
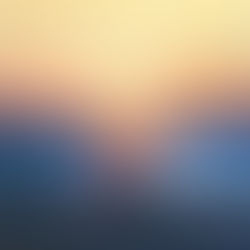
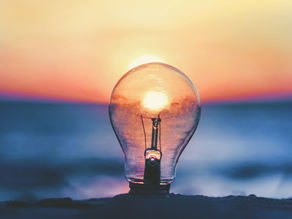
-
May 27, 20223 min read
Future of Mining - The Diversity Imperative for Mining in a Post Pandemic World
As a leader in both technology and mining sectors with a focus on health and safety in mines. How have you experienced positive shifts in...


-
Nov 15, 20213 min read
Our Managing Director on how technology is a boon for people across the mining industry
Hello Theodora, welcome to our show. Firstly, we are glad to know that Canaria Technologies has partnered with Rio Tinto globally to roll...
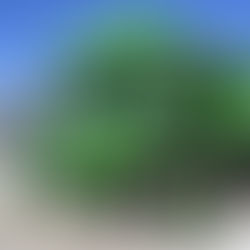
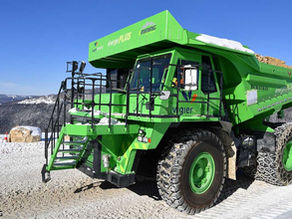
-
Oct 6, 20213 min read
Electrification of Mining Vehicles – An Electric Future
The mining industry has seen electrification climbing the agenda for many years, offering promising opportunities such as cost reduction...
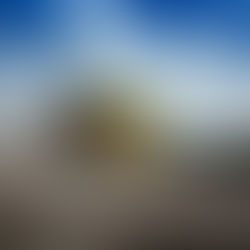
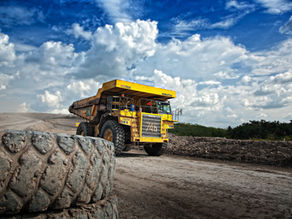
-
Sep 9, 20214 min read
Driving Digital Innovation with AI Through Mining and Industry
As one of the oldest and most labour-intensive industries, it’s not really surprising that digital innovations are not as quickly adopted...
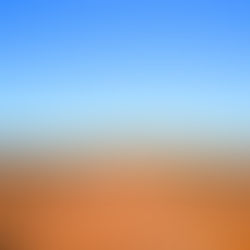
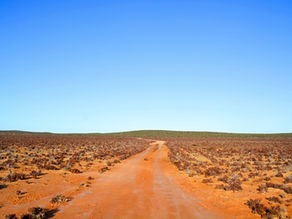
-
Aug 17, 20213 min read
Impacts of Heat Exposure on Productivity Outcomes
Driving economic success is labour productivity, with economists focusing large amounts of attention on understanding, measuring, and...
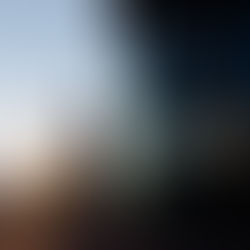
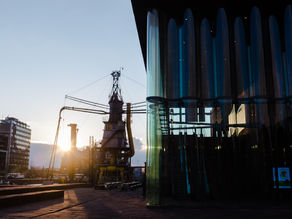
-
Jul 30, 20213 min read
Mining & Industry 4.0 – The Complete Digital Transformation
Mining has seen many challenges over the last 100 years, with the most recent impact being the COVID-19 pandemic - completely...
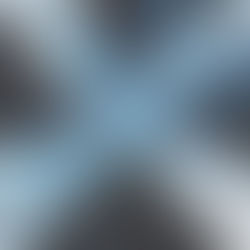
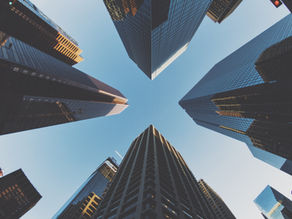
-
Jul 20, 20213 min read
Delivering Holistic Resilience for Post Pandemic Growth
Supercharging companies to pivot through the COVID-19 pandemic has been driven by digitisation, automation and a change in organisational...
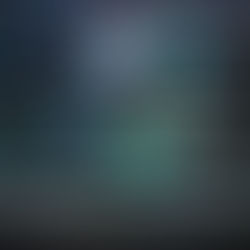
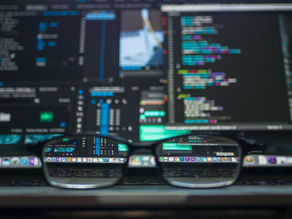
-
Jul 6, 20213 min read
How digital agility is transforming business during COVID-19 and beyond.
For over a year, the COVID-19 pandemic has challenged and disrupted organisations across the globe, with many businesses unable to pivot...
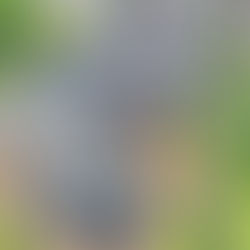
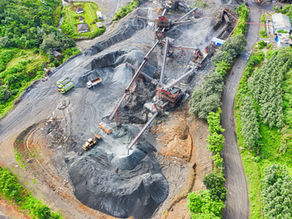
-
Jun 17, 20213 min read
The Importance of ESG Reporting Across Mining and Resources
ESG (environmental, social & governance) has come to fruition through investors and stakeholders alike demanding a shift in attention...
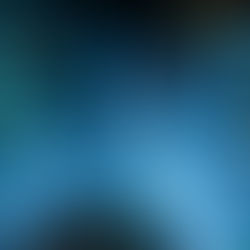

Amy Challenger
Jun 14, 20212 min read
How AI is Ensuring Worker Safety in Every Industry
In recent years, artificial intelligence (AI) has been poised to lead rapid transformation across industries. Most adopters are using AI...
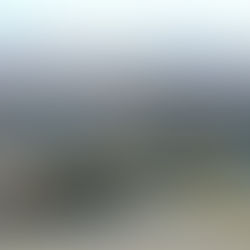
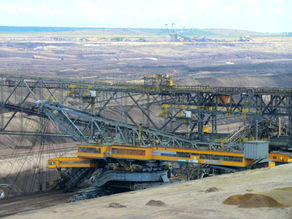
-
Jun 4, 20214 min read
Digital Innovation and Safety Technology Transforming the Mining Industry
Both the international and domestic world of mining have come a long way regarding safety, with the number of total deaths dropping to an...
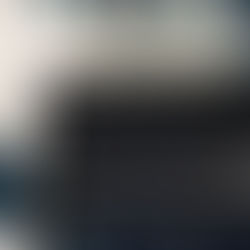
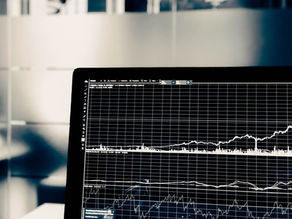
-
May 13, 20214 min read
What is a Predictive Biometric System?
A predictive biometric system combines data about how the human body functions with AI to predict future body functions. It is an...
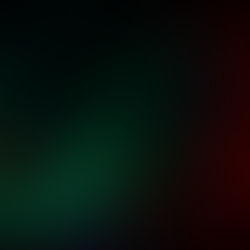
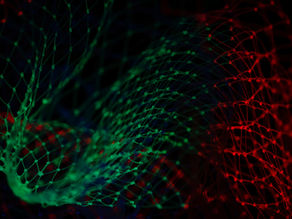
-
May 13, 20212 min read
Machine Learning, Deep Learning and Artificial Intelligence
Last time we covered the definition of AI. You’ll probably have heard the terms AI and ML being used interchangeably in the mainstream...
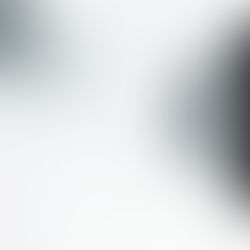
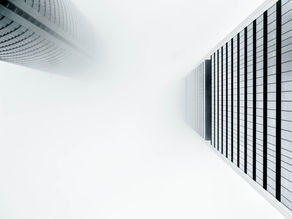
-
Apr 19, 20213 min read
How does AI (Artificial Intelligence) actually work?
The term ‘Artificial Intelligence’ refers not to any one specific technique, but any coding technique that results in the imitation of...
bottom of page